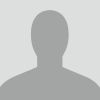
Andrew O. Mellinger
Featured in:
Articles
-
Sep 30, 2024 |
insights.sei.cmu.edu | Julie Cohen |Michael Konrad |Melissa Ludwick |Andrew O. Mellinger
Understanding and evaluating your artificial intelligence (AI) system’s predictions can be challenging. AI and machine learning (ML) classifiers are subject to limitations caused by a variety of factors, including concept or data drift, edge cases, the natural uncertainty of ML training outcomes, and emerging phenomena unaccounted for in training data. These types of factors can lead to bias in a classifier’s predictions, compromising decisions made based on those predictions.
Try JournoFinder For Free
Search and contact over 1M+ journalist profiles, browse 100M+ articles, and unlock powerful PR tools.
Start Your 7-Day Free Trial →