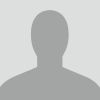
Charles Ellis
Articles
-
Apr 25, 2024 |
biorxiv.org | Masoud Seraji |Charles Ellis |Mohammad S.E. Sendi |Robyn Miller
AbstractA common analysis approach for resting state functional magnetic resonance imaging (rs-fMRI) dynamic functional network connectivity (dFNC) data involves clustering windowed correlation time-series and assigning time windows to clusters (i.e., states) that can be quantified to summarize aspects of the dFNC dynamics. However, those methods can be dominated by a select few features and obscure key dynamics related to less dominant features.
-
Mar 21, 2024 |
biorxiv.org | Charles Ellis |Martina Lapera Sancho |Robyn Miller |Vince Calhoun
AbstractDeep learning methods are increasingly being applied to raw electroencephalogram (EEG) data. However, if these models are to be used in clinical or research contexts, methods to explain them must be developed, and if these models are to be used in research contexts, methods for combining explanations across large numbers of models must be developed to counteract the inherent randomness of existing training approaches.
-
Feb 13, 2024 |
biorxiv.org | Charles Ellis |Robyn Miller |Vince Calhoun |Martina Lapera
AbstractThe diagnosis of schizophrenia (SZ) can be challenging due to its diverse symptom presentation. As such, many studies have sought to identify diagnostic biomarkers of SZ using explainable machine learning methods. However, the generalizability of identified biomarkers in many machine learning-based studies is highly questionable given that most studies only analyze explanations from a small number of models.
-
Dec 18, 2023 |
biorxiv.org | Charles Ellis |Robyn Miller |Vince Calhoun
AbstractWhile deep learning methods are increasingly applied in research contexts for neuropsychiatric disorder diagnosis, small dataset size limits their potential for clinical translation. Data augmentation (DA) could address this limitation, but the utility of EEG DA methods remains relatively underexplored in neuropsychiatric disorder diagnosis. In this study, we train a model for major depressive disorder diagnosis. We then evaluate the utility of 6 EEG DA approaches.
-
Nov 16, 2023 |
biorxiv.org | Charles Ellis |Robyn Miller |Vince Calhoun
AbstractTransfer learning offers a route for developing robust deep learning models on small raw electroencephalography (EEG) datasets. Nevertheless, the utility of applying representations learned from large datasets with a lower sampling rate to smaller datasets with higher sampling rates remains relatively unexplored.
Try JournoFinder For Free
Search and contact over 1M+ journalist profiles, browse 100M+ articles, and unlock powerful PR tools.
Start Your 7-Day Free Trial →