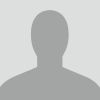
Charlie Saillard
Articles
-
Dec 30, 2024 |
nature.com | Charlie Saillard |Philipp Mann |Charles Maussion |Niklas Klümper |Johannes Breyer |Ralph E. Wirtz | +9 more
Pathogenic activating mutations in the fibroblast growth factor receptor 3 (FGFR3) drive disease maintenance and progression in urothelial cancer. 10–15% of muscle-invasive and metastatic urothelial cancer (MIBC/mUC) are FGFR3-mutant. Selective targeting of FGFR3 hotspot mutations with tyrosine kinase inhibitors (e.g., erdafitinib) is approved for mUC and requires FGFR3 mutational testing. However, current testing assays (polymerase chain reaction or next-generation sequencing) necessitate high tissue quality, have long turnover time, and are expensive. To overcome these limitations, we develop a deep-learning model that detects FGFR3 mutations using routine hematoxylin-eosin slides. Encompassing 1222 cases, our study is a large-scale validation of a model prescreening FGFR3 mutations for MIBC and mUC patients. In this work, we demonstrate that our model achieves high sensitivity (>93%) on advanced and metastatic cases while reducing molecular testing by 40% on average, thereby offering a cost-effective and rapid pre-screening tool for identifying patients eligible for FGFR3 targeted therapies. Detecting FGFR3-mutant muscle-invasive and metastatic urothelial cancers (MIBC/mUC) for targeted therapy remains challenging, but clinically important. Here, the authors develop a deep-learning model to detect FGFR3 mutations in MIBC/mUC from routine histopathology slides, allowing for highly sensitive, rapid, and cost-effective pre-screening.
Try JournoFinder For Free
Search and contact over 1M+ journalist profiles, browse 100M+ articles, and unlock powerful PR tools.
Start Your 7-Day Free Trial →