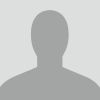
Chris Latimer
Articles
-
Oct 28, 2024 |
producthunt.com | Chris Latimer
HomeProductVectorizeBuild RAG pipelines that are optimized for your data. Vectorize is a data platform for retrieval augmented generation (RAG). It combines RAG evaluation to identify the best way to vectorize your data with a cloud-scale RAG pipeline engine. Vectorize populates your vector database and keeps your vector data fresh. Maker ShoutoutsWe couldn't have built this without... About this launchwas hunted by in Vectorize by SaaS, Developer Tools, Data. Made by , and .
-
Oct 8, 2024 |
morningstar.com | Chris Latimer
BOULDER, Colo., Oct. 08, 2024 (GLOBE NEWSWIRE) -- Vectorize, a pioneering startup in the field of AI-powered data integration, today announced it has raised $3.6 million in seed funding led by True Ventures. The company also unveiled its groundbreaking Retrieval Augmented Generation (RAG) platform designed to transform how businesses of all sizes leverage their data for AI applications.
-
Jun 24, 2024 |
couchbase.com | Chris Latimer |Kiran Matty
For technology leaders and developers, the process of integrating rich, proprietary data into generative AI applications is often filled with challenges. Vector similarity search and retrieval augmented generation are powerful tools to help with this, but one mistake extracting, chunking, or vectorizing your data can mean the difference between an AI agent that performs well and one that frustrates users with poor responses and hallucinations.
-
Jun 16, 2024 |
techsling.com | Chris Latimer
There are three different kinds of RAG, naive, advanced and modular. Large language models (LLMs) have transformed AI, excelling in natural language tasks such as those performed by ChatGPT, Bard, and Claude. These models can generate a wide range of content, from creative writing to complex programming code. Nonetheless, LLMs face issues like hallucinations, outdated information, and opaque, untraceable reasoning processes. It is necessary to know what kind of RAG is being used.
-
Dec 6, 2023 |
dzone.com | Chris Latimer
Building generative AI applications that use retrieval augmented generation (RAG) can pose a host of challenges. Let’s look at troubleshooting RAG implementations that rely on vector databases to retrieve relevant context that’s then included in a prompt to a large language model to provide more relevant results. We will break this process down into two main parts.
Try JournoFinder For Free
Search and contact over 1M+ journalist profiles, browse 100M+ articles, and unlock powerful PR tools.
Start Your 7-Day Free Trial →