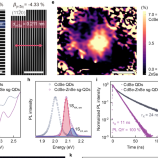
Articles
-
Nov 18, 2024 |
nature.com | Yun S. Song |Heon-Jeong Lee |Chul-Hyun Cho |Jae Kyoung Kim |Dongju Lim |Jung-Been Lee | +3 more
Wearable devices enable passive collection of sleep, heart rate, and step-count data, offering potential for mood episode prediction in mood disorder patients. However, current models often require various data types, limiting real-world application. Here, we develop models that predict future episodes using only sleep-wake data, easily gathered through smartphones and wearables when trained on an individual’s sleep-wake history and past mood episodes. Using mathematical modeling to longitudinal data from 168 patients (587 days average clinical follow-up, 267 days wearable data), we derived 36 sleep and circadian rhythm features. These features enabled accurate next-day predictions for depressive, manic, and hypomanic episodes (AUCs: 0.80, 0.98, 0.95). Notably, daily circadian phase shifts were the most significant predictors: delays linked to depressive episodes, advances to manic episodes. This prospective observational cohort study (ClinicalTrials.gov: NCT03088657, 2017-3-23) shows sleep-wake data, combined with prior mood episode history, can effectively predict mood episodes, enhancing mood disorder management.
Try JournoFinder For Free
Search and contact over 1M+ journalist profiles, browse 100M+ articles, and unlock powerful PR tools.
Start Your 7-Day Free Trial →