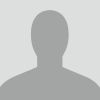
Luca M. Ghiringhelli
Articles
-
Jan 15, 2025 |
pubs.rsc.org | Daniel Speckhard |Humboldt-Universität zu Berlin |Luca M. Ghiringhelli |Tim Bechtel
Big data has ushered in a new wave of predictive power using machine-learning models. In this work, we assess what big means in the context of typical materials-science machine-learning problems. This concerns not only data volume, but also data quality and veracity as much as infrastructure issues.
-
Oct 12, 2023 |
arxiv.org | Luca M. Ghiringhelli
[Submitted on 12 Oct 2023 (v1), last revised 1 Mar 2024 (this version, v3)] Title:Emergence of Latent Binary Encoding in Deep Neural Network Classifiers Download a PDF of the paper titled Emergence of Latent Binary Encoding in Deep Neural Network Classifiers, by Luigi Sbail\`o and Luca Ghiringhelli Download PDF HTML (experimental) Abstract:We investigate the emergence of binary encoding within the latent space of deep-neural-network classifiers.
-
Sep 14, 2023 |
nature.com | Luca M. Ghiringhelli |Carsten Baldauf |Tristan Bereau |Christian Carbogno |Stefano Curtarolo |Claudia Draxl | +9 more
The expansive production of data in materials science, their widespread sharing and repurposing requires educated support and stewardship. In order to ensure that this need helps rather than hinders scientific work, the implementation of the FAIR-data principles (Findable, Accessible, Interoperable, and Reusable) must not be too narrow.
-
Jun 24, 2023 |
nature.com | Matthias Scheffler |Luca M. Ghiringhelli
AbstractReliable artificial-intelligence models have the potential to accelerate the discovery of materials with optimal properties for various applications, including superconductivity, catalysis, and thermoelectricity. Advancements in this field are often hindered by the scarcity and quality of available data and the significant effort required to acquire new data.
Try JournoFinder For Free
Search and contact over 1M+ journalist profiles, browse 100M+ articles, and unlock powerful PR tools.
Start Your 7-Day Free Trial →