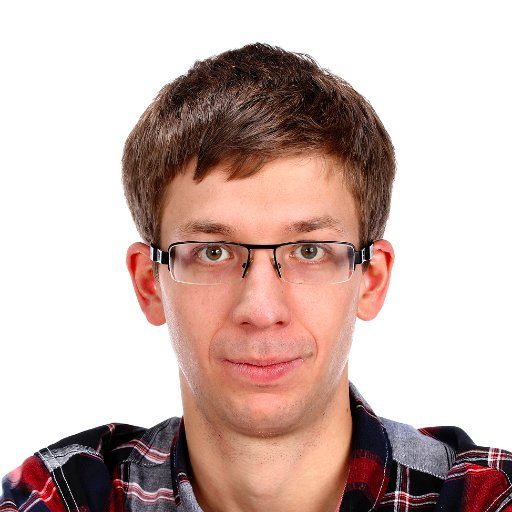
Matei Zaharia
Chief Technology Officer and Co-Founder at Databricks
CTO at @Databricks and CS prof at @UCBerkeley. Working on data+AI, including @ApacheSpark, @DeltaLakeOSS, @MLflow, https://t.co/94gROE5Xa0. https://t.co/nmRYAKG0LZ
Articles
-
2 months ago |
databricks.com | Dipendra Misra |Matei Zaharia |Emanuel Zgraggen |Ta-Chung Chi
Summary: LLMs have revolutionized software development by increasing the productivity of programmers. However, despite off-the-shelf LLMs being trained on a significant amount of code, they are not perfect. One key challenge for our Enterprise customers is the need to perform data intelligence, i.e., to adapt and reason using their own organization’s data. This includes being able to use organization-specific coding concepts, knowledge, and preferences.
-
Jan 29, 2025 |
arxiv.org | Jan Luca |Matei Zaharia |Christopher Potts |Gustavo Alonso
arXivLabs is a framework that allows collaborators to develop and share new arXiv features directly on our website. Both individuals and organizations that work with arXivLabs have embraced and accepted our values of openness, community, excellence, and user data privacy. arXiv is committed to these values and only works with partners that adhere to them. Have an idea for a project that will add value for arXiv's community? Learn more about arXivLabs.
-
Nov 13, 2024 |
databricks.com | Naveen Rao |Matei Zaharia |Patrick Wendell |Eric Peter
Monolithic to ModularThe proof of concept (POC) of any new technology often starts with large, monolithic units that are difficult to characterize. By definition, POCs are designed to show that a technology works without considering issues around extensibility, maintenance, and quality. However, once technologies achieve maturity and are deployed widely, these needs drive product development to be broken down into smaller, more manageable units.
-
Oct 8, 2024 |
databricks.com | Quinn Leng |Jacob P. Portes |Sam Havens |Matei Zaharia
Retrieval Augmented Generation (RAG) is the top use case for Databricks customers who want to customize AI workflows on their own data. The pace of large language model releases is incredibly fast, and many of our customers are looking for up-to-date guidance on how to build the best RAG pipelines. In a previous blog post, we ran over 2,000 long context RAG experiments on 13 popular open source and commercial LLMs to uncover their performance on various domain-specific datasets.
-
Oct 2, 2024 |
databricks.com | Linqing Liu |Matthew Hayes |Ritendra Datta |Matei Zaharia
IntroductionApplying Large Language Models (LLMs) for code generation is becoming increasingly prevalent, as it helps you code faster and smarter. A primary concern with LLM-generated code is its correctness. Most open-source coding benchmarks are designed to evaluate general coding skills. But, in enterprise environments, the LLMs must be capable not only of general programming but also of utilizing domain-specific libraries and tools, such as MLflow and Spark SQL.
Try JournoFinder For Free
Search and contact over 1M+ journalist profiles, browse 100M+ articles, and unlock powerful PR tools.
Start Your 7-Day Free Trial →X (formerly Twitter)
- Followers
- 42K
- Tweets
- 2K
- DMs Open
- No

RT @DbrxMosaicAI: We’re proud to be a platinum sponsor of #MLSys2025 alongside our co-founder & CTO @matei_zaharia serving as general chai…

Super excited about our agreement to acquire @neondatabase, bringing state-of-the-art, serverless elastic Postgres to Databricks! Building end-to-end data and AI apps is about to get much easier.

I am super excited to announce that we have agreed to acquire Neon, a developer-centric serverless Postgres company. The Neon team engineered a new database architecture that offers speed, elastic scaling, and branching and forking. The capabilities that make Neon great for

RT @nikitabase: I’m excited to share a major milestone: Neon is joining forces with @databricks. https://t.co/QZPfL5SDsT Together, we wil…