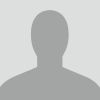
Articles
-
3 weeks ago |
nature.com | Michael Moritz |Eric J. Topol |Pranav Rajpurkar
Decentralized yet coordinated networks of specialized artificial intelligence agents, multi-agent systems for healthcare (MASH), that excel in performing tasks in an assistive or autonomous manner within specific clinical and operational domains are likely to become the next paradigm in medical artificial intelligence.
-
Mar 19, 2024 |
nature.com | Oishi Banerjee |Nikhil Agarwal |Alex Moehring |Feiyang Yu |Pranav Rajpurkar |Tobias Salz
The integration of artificial intelligence (AI) in medical image interpretation requires effective collaboration between clinicians and AI algorithms. Although previous studies demonstrated the potential of AI assistance in improving overall clinician performance, the individual impact on clinicians remains unclear. This large-scale study examined the heterogeneous effects of AI assistance on 140 radiologists across 15 chest X-ray diagnostic tasks and identified predictors of these effects. Surprisingly, conventional experience-based factors, such as years of experience, subspecialty and familiarity with AI tools, fail to reliably predict the impact of AI assistance. Additionally, lower-performing radiologists do not consistently benefit more from AI assistance, challenging prevailing assumptions. Instead, we found that the occurrence of AI errors strongly influences treatment outcomes, with inaccurate AI predictions adversely affecting radiologist performance on the aggregate of all pathologies and on half of the individual pathologies investigated. Our findings highlight the importance of personalized approaches to clinician–AI collaboration and the importance of accurate AI models. By understanding the factors that shape the effectiveness of AI assistance, this study provides valuable insights for targeted implementation of AI, enabling maximum benefits for individual clinicians in clinical practice. A large-scale study examined the heterogeneous effects of artificial intelligence (AI) assistance on 140 radiologists across 15 chest X-ray diagnostic tasks and found that conventional experience-based factors, such as years of experience, subspecialty and familiarity with AI tools, fail to reliably predict the impact of AI assistance.
Try JournoFinder For Free
Search and contact over 1M+ journalist profiles, browse 100M+ articles, and unlock powerful PR tools.
Start Your 7-Day Free Trial →