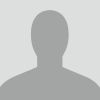
Spotify Engineering
Articles
-
Jun 6, 2024 |
research.atspotify.com | Spotify Engineering
tldr – The wide variety of reasons people listen to music include both individual motivations, like self-awareness and mood regulation, as well as social motivations, such as demonstrating belonging to a group or feeling connected to friends. Music recommendation systems implicitly tend to focus on individual motivations for music listening.
-
May 28, 2024 |
engineering.atspotify.com | Spotify Engineering
Check out Data Platform Explained Part I, where we started sharing the journey of building a data platform, its building blocks, and the motivation for investing into building a platformized solution at Spotify. In Data Platform Explained Part I, we shared the first steps in the journey to build a data platform, the insights that indicate it’s time to start building one, and how we are organized to succeed on it.
-
Apr 30, 2024 |
engineering.atspotify.com | Spotify Engineering |Pia Nilsson
Today, we announced Spotify’s latest products and services for companies adopting Backstage, the open source framework for building internal developer portals (IDPs). Whether your company needs a highly customized IDP built from scratch or an out-of-the-box solution that’s ready to go ASAP, we want to make it easy for anyone to maximize the value they get from their Backstage developer portal.
-
Jul 29, 2023 |
research.atspotify.com | Spotify Engineering
Reinforcement learning (RL) is an established tool for sequential decision making. In this work, we apply RL to solve an automatic music playlist generation problem. In particular, we developed a RL framework for set sequencing that optimizes for user satisfaction metrics via the use of a simulated playlist-generation environment.
-
Mar 23, 2023 |
research.atspotify.com | Spotify Engineering
Goal-setting in recommender systemsRecommender systems typically look to users’ past consumption to predict what they may want next. In practice, this approach tends to work best when what the user wants is similar to what they have consumed recently, and when it is relatively easy for that person to evaluate new items. On the other hand, this approach works less well when what the user wants is something different and potentially something that is not captured by their past consumption [1].
Try JournoFinder For Free
Search and contact over 1M+ journalist profiles, browse 100M+ articles, and unlock powerful PR tools.
Start Your 7-Day Free Trial →