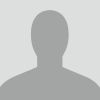
Thanasis Pittas
Articles
-
Jan 9, 2025 |
arxiv.org | Daniel Kane |Sihan Liu |Thanasis Pittas
arXivLabs is a framework that allows collaborators to develop and share new arXiv features directly on our website. Both individuals and organizations that work with arXivLabs have embraced and accepted our values of openness, community, excellence, and user data privacy. arXiv is committed to these values and only works with partners that adhere to them. Have an idea for a project that will add value for arXiv's community? Learn more about arXivLabs.
-
Mar 15, 2024 |
arxiv.org | Daniel Kane |Sushrut Karmalkar |Ankit Pensia |Thanasis Pittas
-
Dec 19, 2023 |
arxiv.org | Jasper C. H |Daniel Kane |Thanasis Pittas
-
Jun 22, 2023 |
arxiv.org | Daniel Kane |Thanasis Pittas |Nikos Zarifis
arXiv:2306.13057 (cs) Download a PDF of the paper titled SQ Lower Bounds for Learning Bounded Covariance GMMs, by Ilias Diakonikolas and 3 other authors Download PDF Submission history From: Thanasis Pittas [ view email] [v1] Thu, 22 Jun 2023 17:23:36 UTC (35 KB) Bibliographic Tools Bibliographic Explorer Toggle Bibliographic Explorer () Litmaps Toggle Litmaps (What is Litmaps?) scite.ai Toggle scite Smart Citations (What are Smart Citations?) Code, Data, Media Links to Code Toggle CatalyzeX...
-
May 4, 2023 |
arxiv.org | Daniel Kane |Ankit Pensia |Thanasis Pittas
We study principal component analysis (PCA), where given a dataset in $\mathbb{R}^d$ from a distribution, the task is to find a unit vector $v$ that approximately maximizes the variance of the distribution after being projected along $v$. Despite being a classical task, standard estimators fail drastically if the data contains even a small fraction of outliers, motivating the problem of robust PCA.
Try JournoFinder For Free
Search and contact over 1M+ journalist profiles, browse 100M+ articles, and unlock powerful PR tools.
Start Your 7-Day Free Trial →