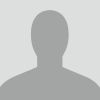
Xiaoshan Luo
Articles
-
Nov 11, 2024 |
nature.com | Xiaoshan Luo |Zhenyu Wang |Jian Lv |Yanchao Wang
AbstractRecent advances in deep learning generative models (GMs) have created high capabilities in accessing and assessing complex high-dimensional data, allowing superior efficiency in navigating vast material configuration space in search of viable structures. Coupling such capabilities with physically significant data to construct trained models for materials discovery is crucial to moving this emerging field forward.
-
Nov 11, 2024 |
flipboard.com | Xiaoshan Luo |Zhenyu Wang |Pengyue Gao |Jian Lv |Yanchao Wang |Changfeng Chen | +1 more
1 hour agoParents of AI nude photo victims call for resignationsAn attorney for families of students at Lancaster Country Day School are demanding the school's administrator and board president resign following an investigation into artificial intelligence-generated nude images. 3 hours agoLegacy of C Programming Language. As a member of the current generation, learning C and then C++ is a rite of passage we all must go through in our college years.
-
Mar 22, 2024 |
link.aps.org | Zhenyu Wang |Xiaoyang Wang |Xiaoshan Luo |Pengyue Gao
Crystal structure prediction (CSP) and machine learning potential (MLP) are two fundamental methods for modern computational material discovery. While the former aims at efficient sampling of the potential energy surface (PES) for discovering new materials, the latter focuses on reproducing the PES to accelerate various atomic simulation tasks. In this work, we combine the two methods within a concurrent learning framework in an effort to generate efficient MLP models for accelerating CSP.
Try JournoFinder For Free
Search and contact over 1M+ journalist profiles, browse 100M+ articles, and unlock powerful PR tools.
Start Your 7-Day Free Trial →