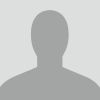
A. Longhena
Articles
-
Jul 18, 2024 |
pubs.aip.org | Johns Hopkins |Thomas Varley |A. Longhena
Transformations are a key tool in the qualitative study of dynamical systems: transformations to a normal form, for example, underpin the study of instabilities and bifurcations. In this work, we test, and when possible establish, an equivalence between two different artificial neural networks by attempting to construct a data-driven transformation between them, using diffusion maps with a Mahalanobis-like metric.
-
Jul 12, 2024 |
pubs.aip.org | Basque Country |Thomas Varley |John McDonough |A. Longhena
We study the properties of Lévy flights with index at elapsed times smaller than those required for reaching the diffusive limit, and we focus on the bulk of the walkers’ distribution rather than on its tails.
-
Jul 10, 2024 |
pubs.aip.org | Thomas Varley |John McDonough |A. Longhena |Hauke Kraemer
Phase space reconstruction (PSR) methods allow for the analysis of low-dimensional data with methods from dynamical systems theory, but their application to prediction models, such as those from machine learning (ML), is limited. Therefore, we here present a model adaptive phase space reconstruction (MAPSR) method that unifies the process of PSR with the modeling of the dynamical system. MAPSR is a differentiable PSR based on time-delay embedding and enables ML methods for modeling.
-
Jul 9, 2024 |
pubs.aip.org | Thomas Varley |A. Longhena |John McDonough
In this paper, we introduce an efficient method for identifying fractional dynamic systems using extended sparse regression and cross-validation techniques. The former identifies equations that fit the data with varying candidate functions, while the latter determines the optimal equation with the fewest terms yet ensuring accuracy. The identified optimal equation is expected to share the same dynamic properties as the original fractional system.
-
Jul 9, 2024 |
pubs.aip.org | Thomas Varley |A. Longhena |John McDonough
Neural networks are popular data-driven modeling tools that come with high data collection costs. This paper proposes a residual-based multipeaks adaptive sampling (RMAS) algorithm, which can reduce the demand for a large number of samples in the identification of stochastic dynamical systems. Compared to classical residual-based sampling algorithms, the RMAS algorithm achieves higher system identification accuracy without relying on any hyperparameters.
Try JournoFinder For Free
Search and contact over 1M+ journalist profiles, browse 100M+ articles, and unlock powerful PR tools.
Start Your 7-Day Free Trial →