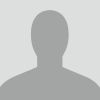
Bart Bussmann
Articles
-
Aug 23, 2024 |
lesswrong.com | Bart Bussmann |Michael Pearce |Patrick Leask |Joseph Bloom
Bart, Michael and Patrick are joint first authors. Research conducted as part of MATS 6.0 in Lee Sharkey and Neel Nanda’s streams. Thanks to Mckenna Fitzgerald and Robert Krzyzanowski for their feedback!TL;DR:Sparse Autoencoder (SAE) latents have been shown to typically be monosemantic (i.e. correspond to an interpretable property of the input). It is sometimes implicitly assumed that they are therefore atomic, i.e. simple, irreducible units that make up the model’s computation.
-
Aug 16, 2024 |
lesswrong.com | Patrick Leask |Bart Bussmann |Neel Nanda
TL;DR: We demonstrate that the decoder directions of GPT-2 SAEs are highly structured by finding a historical date direction onto which projecting non-date related features lets us read off their historical time period by comparison to year features. Calendar years are linear: there are as many years between 2000 and 2024, as there are between 1800 and 1824. Linear probes can be used to predict years of particular events from the activations of language models.
-
Jul 13, 2024 |
lesswrong.com | Bart Bussmann |Joseph Bloom |Neel Nanda |Curt Tigges
Work done in Neel Nanda’s stream of MATS 6.0, equal contribution by Bart Bussmann and Patrick Leask, Patrick Leask is concurrently a PhD candidate at Durham UniversityTL;DR: When you scale up an SAE, the features in the larger SAE can be categorized in two groups: 1) “novel features” with new information not in the small SAE and 2) “reconstruction features” that sparsify information that already exists in the small SAE. You can stitch SAEs by adding the novel features to the smaller SAE.
Try JournoFinder For Free
Search and contact over 1M+ journalist profiles, browse 100M+ articles, and unlock powerful PR tools.
Start Your 7-Day Free Trial →