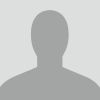
Joseph Bloom
Articles
-
Oct 7, 2024 |
lesswrong.com | Joseph Bloom
In previous work, we found a problematic form a feature splitting called "feature absorption" when analyzing Gemma Scope SAEs. We hypothesized that this was due to SAEs struggling to separate co-occurrence between features, but we did not prove this. In this post, we set up toy models where we can explicitly control feature representations and co-occurrence rates and show the following:Feature absorption happens when features co-occur.
-
Sep 25, 2024 |
lesswrong.com | Joseph Bloom
This research was completed for London AI Safety Research (LASR) Labs 2024. The team was supervised by Joseph Bloom (Decode Research). Find out more about the programme and express interest in upcoming iterations here. This high level summary will be most accessible to those with relevant context including an understanding of SAEs. The importance of this work rests in part on the surrounding , and potential philosophical issues.
-
Aug 23, 2024 |
lesswrong.com | Bart Bussmann |Michael Pearce |Patrick Leask |Joseph Bloom
Bart, Michael and Patrick are joint first authors. Research conducted as part of MATS 6.0 in Lee Sharkey and Neel Nanda’s streams. Thanks to Mckenna Fitzgerald and Robert Krzyzanowski for their feedback!TL;DR:Sparse Autoencoder (SAE) latents have been shown to typically be monosemantic (i.e. correspond to an interpretable property of the input). It is sometimes implicitly assumed that they are therefore atomic, i.e. simple, irreducible units that make up the model’s computation.
-
Jul 13, 2024 |
lesswrong.com | Bart Bussmann |Joseph Bloom |Neel Nanda |Curt Tigges
Work done in Neel Nanda’s stream of MATS 6.0, equal contribution by Bart Bussmann and Patrick Leask, Patrick Leask is concurrently a PhD candidate at Durham UniversityTL;DR: When you scale up an SAE, the features in the larger SAE can be categorized in two groups: 1) “novel features” with new information not in the small SAE and 2) “reconstruction features” that sparsify information that already exists in the small SAE. You can stitch SAEs by adding the novel features to the smaller SAE.
-
Apr 1, 2024 |
lesswrong.com | Callum McDougall |Joseph Bloom
Epistemic status - self-evident. In this post, we interpret a small sample of Sparse Autoencoder features which reveal meaningful computational structure in the model that is clearly highly researcher-independent and of significant relevance to AI alignment. Recent excitement about Sparse Autoencoders (SAEs) has been mired by the following question: Do SAE features reflect properties of the model, or just capture correlational structure in the underlying data distribution?
Try JournoFinder For Free
Search and contact over 1M+ journalist profiles, browse 100M+ articles, and unlock powerful PR tools.
Start Your 7-Day Free Trial →