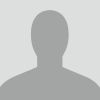
Guillaume Bellegarda
Articles
-
Jun 28, 2024 |
arxiv.org | Guillaume Bellegarda |Auke Ijspeert
[Submitted on 28 Jun 2024] Title:Learning Human-Robot Handshaking Preferences for Quadruped Robots View a PDF of the paper titled Learning Human-Robot Handshaking Preferences for Quadruped Robots, by Alessandra Chappuis and 2 other authors View PDF HTML (experimental) Abstract:Quadruped robots are showing impressive abilities to navigate the real world. If they are to become more integrated into society, social trust in interactions with humans will become increasingly important.
-
Apr 30, 2024 |
thetechstreetnow.com | Guillaume Bellegarda |Milad Shafiee |Auke Ijspeert
26 minutes ago phys.org A four-legged robot trained with machine learning by EPFL researchers has learned to avoid falls by spontaneously switching between walking, trotting, and pronking—a milestone for roboticists as well as biologists interested in animal locomotion.
-
Apr 27, 2024 |
arxiv.org | Milad Shafiee |Peizhuo Li |Guillaume Bellegarda |Auke Ijspeert
[Submitted on 27 Apr 2024] Title:Learning-based Hierarchical Control: Emulating the Central Nervous System for Bio-Inspired Legged Robot Locomotion View a PDF of the paper titled Learning-based Hierarchical Control: Emulating the Central Nervous System for Bio-Inspired Legged Robot Locomotion, by Ge Sun and 5 other authors View PDF HTML (experimental) Abstract:Animals possess a remarkable ability to navigate challenging terrains, achieved through the interplay of various pathways between the...
-
Apr 8, 2024 |
nature.com | Milad Shafiee |Guillaume Bellegarda |Auke Ijspeert
AbstractQuadruped animals are capable of seamless transitions between different gaits. While energy efficiency appears to be one of the reasons for changing gaits, other determinant factors likely play a role too, including terrain properties. In this article, we propose that viability, i.e., the avoidance of falls, represents an important criterion for gait transitions.
-
Aug 21, 2023 |
nature.com | Wanming Yu |Auke Ijspeert |Christopher McGreavy |Eleftherios Triantafyllidis |Guillaume Bellegarda
AbstractRobot motor skills can be acquired by deep reinforcement learning as neural networks to reflect state–action mapping. The selection of states has been demonstrated to be crucial for successful robot motor learning. However, because of the complexity of neural networks, human insights and engineering efforts are often required to select appropriate states through qualitative approaches, such as ablation studies, without a quantitative analysis of the state importance.
Try JournoFinder For Free
Search and contact over 1M+ journalist profiles, browse 100M+ articles, and unlock powerful PR tools.
Start Your 7-Day Free Trial →