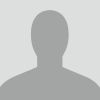
Articles
-
Apr 30, 2024 |
nature.com | Volodymyr Koverga |Au Hai Nguyen |Heng Jiang |Ke Wang |Meng Liao |Jun Chen | +7 more
Extending the lifespan of lithium (Li) batteries involves managing reactions at the Li anode and stabilizing the solid–electrolyte interphase (SEI) through strategic regulation of the electrolyte composition. Here we synthesized a fluorinated cyclic ether with minimized Li-ion coordination capability and enhanced electrochemical stability. We demonstrated its crucial role in manipulating the SEI formation process by differentiating the contribution of dual anions to the SEI layer. Consequently, a bilayer SEI is formed, featuring a Li2O-rich inner layer and a LiF-rich outer layer, enabling improved stability and reversibility of Li-metal anodes. The developed electrolyte shows remarkable improvement in calendar life and cycling stability of Li (50 µm)||NMC811 (4 mAh cm−2) cells, maintaining 80% capacity after 568 and 218 cycles at room temperature and 60 °C, respectively. Furthermore, our 410 Wh kg−1 prototype pouch cells demonstrate 80% capacity retention for 470 cycles. Lithium battery performance hinges significantly on the solvation structure of the electrolyte and the resulting interphase. Here the authors introduce a fluorinated ether with minimal lithium-ion coordination and enhanced electrochemical stability, thus improving both cyclability and calendar life.
-
Apr 25, 2024 |
nature.com | Bo Zhang |Xun Xu |Jinliuxing Yang |Yuan Liu |Zhuoting Zhu |Jun Chen | +8 more
To develop and validate a machine learning based algorithm to estimate physical activity (PA) intensity using the smartwatch with the capacity to record PA and determine outdoor state. Two groups of participants, including 24 adults (13 males) and 18 children (9 boys), completed a sequential activity trial. During each trial, participants wore a smartwatch, and energy expenditure was measured using indirect calorimetry as gold standard. The support vector machine algorithm and the least squares regression model were applied for the metabolic equivalent (MET) estimation using raw data derived from the smartwatch. Exercise intensity was categorized based on MET values into sedentary activity (SED), light activity (LPA), moderate activity (MPA), and vigorous activity (VPA). The classification accuracy was evaluated using area under the ROC curve (AUC). The METs estimation accuracy were assessed via the mean absolute error (MAE), the correlation coefficient, Bland–Altman plots, and intraclass correlation (ICC). A total of 24 adults aged 21–34 years and 18 children aged 9–13 years participated in the study, yielding 1790 and 1246 data points for adults and children respectively for model building and validation. For adults, the AUC for classifying SED, MVPA, and VPA were 0.96, 0.88, and 0.86, respectively. The MAE between true METs and estimated METs was 0.75 METs. The correlation coefficient and ICC were 0.87 (p < 0.001) and 0.89, respectively. For children, comparable levels of accuracy were demonstrated, with the AUC for SED, MVPA, and VPA being 0.98, 0.89, and 0.85, respectively. The MAE between true METs and estimated METs was 0.80 METs. The correlation coefficient and ICC were 0.79 (p < 0.001) and 0.84, respectively. The developed model successfully estimated PA intensity with high accuracy in both adults and children. The application of this model enables independent investigation of PA intensity, facilitating research in health monitoring and potentially in areas such as myopia prevention and control.
Try JournoFinder For Free
Search and contact over 1M+ journalist profiles, browse 100M+ articles, and unlock powerful PR tools.
Start Your 7-Day Free Trial →