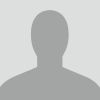
Lee Sharkey
Articles
-
2 months ago |
lesswrong.com | Lee Sharkey
This review collects the perspectives of its various authors and represents a synthesis of their views by Apollo Research on behalf of Schmidt Sciences. The perspectives presented here do not necessarily reflect the views of any individual author or the institutions with which they are affiliated. Mechanistic interpretability aims to understand the computational mechanisms underlying neural networks’ capabilities in order to accomplish concrete scientific and engineering goals.
-
2 months ago |
lesswrong.com | Lucius Bushnaq |Dan Braun |Stefan Hex |Lee Sharkey
This is a linkpost for Apollo Research's new interpretability paper: "Interpretability in Parameter Space: Minimizing Mechanistic Description Length with Attribution-based Parameter Decomposition". We introduce a new method for directly decomposing neural network parameters into mechanistic components. At Apollo, we've spent a lot of time thinking about how the computations of neural networks might be structured, and how those computations might be embedded in networks' parameters.
-
Jul 18, 2024 |
lesswrong.com | Lee Sharkey |Lucius Bushnaq |Dan Braun |Stefan Hex
Why we made this list: The interpretability team at Apollo Research wrapped up a few projects recently. In order to decide what we’d work on next, we generated a lot of different potential projects. Unfortunately, we are computationally bounded agents, so we can't work on every project idea that we were excited about! Previous lists of project ideas (such as Neel’s collation of 200 Concrete Open Problems in Mechanistic Interpretability) have been very useful for people breaking into the field.
-
Jul 2, 2024 |
lesswrong.com | Lee Sharkey
This work was produced as part of Lee Sharkey's stream in the ML Alignment & Theory Scholars Program - Winter 2023-24 CohortSparse dictionary learning (SDL) has attracted a lot of attention recently as a method for interpreting transformer activations. They demonstrate that model activations can often be explained using a sparsely-activating, overcomplete set of human-interpretable directions.
-
May 29, 2024 |
lesswrong.com | Marius Hobbhahn |Lee Sharkey |Lucius Bushnaq |Dan Braun
This is a linkpost for: www.apolloresearch.ai/blog/the-first-year-of-apollo-researchApollo Research is an evaluation organization focusing on risks from deceptively aligned AI systems. We conduct technical research on AI model evaluations and interpretability and have a small AI governance team. As of 29 May 2024, we are one year old. For the UK AI Safety Summit, we developed a demonstration that Large Language Models (LLMs) can strategically deceive their primary users when put under pressure.
Try JournoFinder For Free
Search and contact over 1M+ journalist profiles, browse 100M+ articles, and unlock powerful PR tools.
Start Your 7-Day Free Trial →