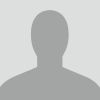
Lucius Bushnaq
Articles
-
Jan 25, 2025 |
lesswrong.com | Lucius Bushnaq |Dan Braun |Stefan Hex |Lee Sharkey
This is a linkpost for Apollo Research's new interpretability paper: "Interpretability in Parameter Space: Minimizing Mechanistic Description Length with Attribution-based Parameter Decomposition". We introduce a new method for directly decomposing neural network parameters into mechanistic components. At Apollo, we've spent a lot of time thinking about how the computations of neural networks might be structured, and how those computations might be embedded in networks' parameters.
-
Jan 8, 2025 |
lesswrong.com | Lucius Bushnaq |Daniel Murfet |Joseph Miller |Matthew Clarke
TL;DR: There may be a fundamental problem with interpretability work that attempts to understand neural networks by decomposing their individual activation spaces in isolation: It seems likely to find features of the activations - features that help explain the statistical structure of activation spaces, rather than features of the model - the features the model’s own computations make use of.
-
Dec 7, 2024 |
lesswrong.com | Lucius Bushnaq
Note: This is a more fleshed-out version of this post and includes theoretical arguments justifying the empirical findings. If you've read that one, feel free to skip to the proofs.
-
Dec 7, 2024 |
lesswrong.com | Lucius Bushnaq |Dmitry Vaintrob
TL:DR: Recently, Lucius held a presentation on the nature of deep learning and why it generalises to new data. Kaarel, Dmitry and Lucius talked about the slides for that presentation in a group chat. The conversation quickly became a broader discussion on the nature of intelligence and how much we do or don't know about it. Lucius: I recently held a small talk presenting an idea for how and why deep learning generalises.
-
Oct 26, 2024 |
lesswrong.com | Lucius Bushnaq |Stefan Heimersheim |Daniel Murfet
Epistemic status: Low confidence. This is a bit of an experiment in lowering my bar for what I make a proper post instead of a shortform. It's just an early stage idea that might go nowhere. It looks to me like neural networks might naturally develop error-correction mechanisms because error correction dramatically increases the volume of solutions in the loss landscape. In other words, neural networks with error correction seem like they’d tend to have much lower learning coefficients.
Try JournoFinder For Free
Search and contact over 1M+ journalist profiles, browse 100M+ articles, and unlock powerful PR tools.
Start Your 7-Day Free Trial →