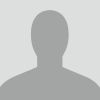
Matthew VanDyke
Articles
-
Dec 6, 2024 |
nature.com | Md Hakimul Haque |Wanyun Shao |Matthew VanDyke |Feng Hao |Hemal Dey
Machine learning (ML) models can simulate flood risk by identifying critical non-linear relationships between flood damage locations and flood risk factors (FRFs). To explore it, Tampa Bay, Florida, is selected as a test site. The study’s goal is to simulate flood risk and identify dominant FRFs using historical flood damage data as target variable, with 16 FRFs as predictor variables. Five different ML models such as decision tree (DT), support vector machine (SVM), adaptive boosting (AdaBoost), extreme gradient boosting (XGBoost), and random forest (RF) were adopted. RF classifies 2.42% of Tampa Bay as very high risk and 2.54% as high risk, while XGBoost classifies 3.85% as very high risk and 1.11% as high risk. Moreover, the communities reside at low altitudes and near the waterbodies, with dense man-made infrastructure, are at high flood risk. This study introduces a comprehensive framework for flood risk assessment and helps policymakers mitigate flood risk.
-
Oct 21, 2024 |
jcom.sissa.it | Matthew VanDyke |Sara K. Yeo
Although the literature in the science of science communication has grown substantially in thepast two decades, and the importance of “setting your goal(s)” and “knowing your audience(s)” iswell-established in practice and in scholarship [Besley & Dudo, 2022; Smith, 2021], more work isneeded to understand and test tactics that science communicators might implement inservice of strategic communication, particularly in the domain of basic science, alsoknown as fundamental or discovery science.
Try JournoFinder For Free
Search and contact over 1M+ journalist profiles, browse 100M+ articles, and unlock powerful PR tools.
Start Your 7-Day Free Trial →