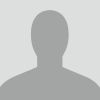
Pulkit Agrawal
Articles
-
Sep 28, 2024 |
agrawal-pulkit.medium.com | Pulkit Agrawal
Pulkit Agrawal·Follow5 min read·--Token Bucket rate LimitingIn today’s fast-paced systems, controlling the rate of incoming requests is crucial to avoid overwhelming your servers. Rate limiting helps regulate the flow of requests, ensuring stability and efficiency. One of the most commonly used algorithms is the Token Bucket Filter. In this blog, we’ll learn how to implement a thread-safe Token Bucket Filter to efficiently handle multiple requests (or threads).
-
May 10, 2024 |
arxiv.org | Idan Shenfeld |Akash Srivastava |Yoon Kim |Pulkit Agrawal
arXivLabs is a framework that allows collaborators to develop and share new arXiv features directly on our website. Both individuals and organizations that work with arXivLabs have embraced and accepted our values of openness, community, excellence, and user data privacy. arXiv is committed to these values and only works with partners that adhere to them. Have an idea for a project that will add value for arXiv's community? Learn more about arXivLabs.
-
Apr 4, 2024 |
arxiv.org | Anthony Simeonov |Pulkit Agrawal |Idan Shenfeld
[Submitted on 4 Apr 2024] Title:JUICER: Data-Efficient Imitation Learning for Robotic Assembly View a PDF of the paper titled JUICER: Data-Efficient Imitation Learning for Robotic Assembly, by Lars Ankile and Anthony Simeonov and Idan Shenfeld and Pulkit Agrawal View PDF HTML (experimental) Abstract:While learning from demonstrations is powerful for acquiring visuomotor policies, high-performance imitation without large demonstration datasets remains challenging for tasks requiring precise,...
-
Oct 31, 2023 |
arxiv.org | Marcel Torne |Zihan Wang |Samedh Desai |Pulkit Agrawal
arXivLabs is a framework that allows collaborators to develop and share new arXiv features directly on our website. Both individuals and organizations that work with arXivLabs have embraced and accepted our values of openness, community, excellence, and user data privacy. arXiv is committed to these values and only works with partners that adhere to them. Have an idea for a project that will add value for arXiv's community? Learn more about arXivLabs.
Parallel $Q$-Learning: Scaling Off-policy Reinforcement Learning under Massively Parallel Simulation
Jul 24, 2023 |
arxiv.org | Tao Chen |Anurag Ajay |Pulkit Agrawal |Zhang-Wei Hong
arXiv:2307.12983 (cs) Download a PDF of the paper titled Parallel $Q$-Learning: Scaling Off-policy Reinforcement Learning under Massively Parallel Simulation, by Zechu Li and 4 other authors Download PDF Submission history From: Tao Chen [ view email] [v1] Mon, 24 Jul 2023 17:59:37 UTC (6,180 KB) Bibliographic Tools Bibliographic Explorer Toggle Bibliographic Explorer () Litmaps Toggle Litmaps (What is Litmaps?) scite.ai Toggle scite Smart Citations (What are Smart Citations?) Code, Data,...
Try JournoFinder For Free
Search and contact over 1M+ journalist profiles, browse 100M+ articles, and unlock powerful PR tools.
Start Your 7-Day Free Trial →