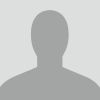
Articles
-
Dec 20, 2023 |
amazon.science | Larry Hardesty |Changyou Chen |Raffay Hamid
Last month, at its annual re:Invent developers’ conference, Amazon Web Services (AWS) announced the release of two new additions to its Titan family of foundation models, both of which translate between text and images. With Amazon Titan Multimodal Embeddings, now available through Amazon Bedrock, customers can upload their own sets of images and then search them using text, related images, or both.
-
Oct 6, 2023 |
amazon.science | Gunnar Sigurdsson |Raffay Hamid |Changyou Chen
Remote-object grounding is the task of automatically determining where in the local environment to find an object specified in natural language.
-
Oct 4, 2023 |
amazon.science | Changyou Chen |Raffay Hamid |Yi Xie
Many recent advances in artificial intelligence are the result of representation learning: a machine learning model learns to represent data items as vectors in a multidimensional space, where geometric relationships between vectors correspond to semantic relationships between items.
-
Aug 22, 2023 |
amazon.science | Austin Xu |Arjun Seshadri |Raghudeep Gadde |Raffay Hamid
Machine learning (ML) models thrive on data, but gathering and labeling training data can be a resource-intensive process. A common way to address this challenge is with synthetic data, but even synthetic data usually requires laborious hand annotation by human analysts. At this year’s Computer Vision and Pattern Recognition conference (CVPR), we presented a method called HandsOff that eliminates the need to hand-annotate synthetic image data.
-
Jun 30, 2023 |
amazon.science | Aviv A. Rosenberg |Dmitry Sotnikov |Raffay Hamid |Tal Lancewick
Policy Optimization (PO) is one of the most popular methods in Reinforcement Learning (RL). Thus, theoretical guarantees for PO algorithms have become especially important to the RL community. In this paper, we study PO in adversarial MDPs with a challenge that arises in almost every real-world application – delayed bandit feedback. We give the first near-optimal regret bounds for PO in tabular MDPs, and may even surpass state-of-the-art (which uses less efficient methods).
Try JournoFinder For Free
Search and contact over 1M+ journalist profiles, browse 100M+ articles, and unlock powerful PR tools.
Start Your 7-Day Free Trial →