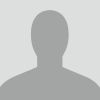
Tal Lancewick
Featured in:
amazon.science
Articles
-
Jun 30, 2023 |
amazon.science | Aviv A. Rosenberg |Dmitry Sotnikov |Raffay Hamid |Tal Lancewick
Policy Optimization (PO) is one of the most popular methods in Reinforcement Learning (RL). Thus, theoretical guarantees for PO algorithms have become especially important to the RL community. In this paper, we study PO in adversarial MDPs with a challenge that arises in almost every real-world application – delayed bandit feedback. We give the first near-optimal regret bounds for PO in tabular MDPs, and may even surpass state-of-the-art (which uses less efficient methods).
Try JournoFinder For Free
Search and contact over 1M+ journalist profiles, browse 100M+ articles, and unlock powerful PR tools.
Start Your 7-Day Free Trial →