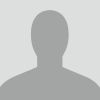
Sijia Huang
Articles
-
Nov 26, 2024 |
nature.com | Zhaojun Zhang |Divij Mathew |Tristan L. Lim |Kaishu Mason |Sijia Huang |E. John Wherry | +5 more
Data integration to align cells across batches has become a cornerstone of single-cell data analysis, critically affecting downstream results. Currently, there are no guidelines for when the biological differences between samples are separable from batch effects. Here we show that current paradigms for single-cell data integration remove biologically meaningful variation and introduce distortion. We present a statistical model and computationally scalable algorithm, CellANOVA (cell state space analysis of variance), that harnesses experimental design to explicitly recover biological signals that are erased during single-cell data integration. CellANOVA uses a ‘pool-of-controls’ design concept, applicable across diverse settings, to separate unwanted variation from biological variation of interest and allow the recovery of subtle biological signals. We apply CellANOVA to diverse contexts and validate the recovered biological signals by orthogonal assays. In particular, we show that CellANOVA is effective in the challenging case of single-cell and single-nucleus data integration, where it recovers subtle biological signals that can be validated and replicated by external data. A statistical framework quantifies single-cell batch variation and recovers meaningful biological signals.
Try JournoFinder For Free
Search and contact over 1M+ journalist profiles, browse 100M+ articles, and unlock powerful PR tools.
Start Your 7-Day Free Trial →