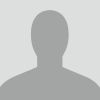
Yanan Wu
Articles
-
Sep 17, 2024 |
dx.doi.org | Yanan Wu |Kun Zhao |Shuai Wu |Yan Su
Physico-Chemical Treatment and Resource RecoverySeptember 17, 2024 Yanan Wu College of Water Resources and Hydropower Engineering, North China Electric Power University, Beijing 102206, China * Kun Zhao College of Water Resources and Hydropower Engineering, North China Electric Power University, Beijing 102206, China College of Environmental Science and Engineering, North China Electric Power University, Beijing 102206, China *Email: [email protected] Shuai Wu Center for Water and Ecology,...
-
Sep 16, 2024 |
mdpi.com | Yonghui Liu |Yanan Wu |Zijian Li |Dong Wan
All articles published by MDPI are made immediately available worldwide under an open access license. No special permission is required to reuse all or part of the article published by MDPI, including figures and tables. For articles published under an open access Creative Common CC BY license, any part of the article may be reused without permission provided that the original article is clearly cited. For more information, please refer to https://www.mdpi.com/openaccess.
-
Jun 14, 2024 |
mdpi.com | Dong Wan |Yanan Wu |Yujun Liu |Yonghui Liu
All articles published by MDPI are made immediately available worldwide under an open access license. No specialpermission is required to reuse all or part of the article published by MDPI, including figures and tables. Forarticles published under an open access Creative Common CC BY license, any part of the article may be reused withoutpermission provided that the original article is clearly cited. For more information, please refer tohttps://www.mdpi.com/openaccess.
-
Jan 11, 2024 |
arxiv.org | Yanan Wu
arXivLabs is a framework that allows collaborators to develop and share new arXiv features directly on our website. Both individuals and organizations that work with arXivLabs have embraced and accepted our values of openness, community, excellence, and user data privacy. arXiv is committed to these values and only works with partners that adhere to them. Have an idea for a project that will add value for arXiv's community? Learn more about arXivLabs.
-
Sep 25, 2023 |
ywu120766.medium.com | Yanan Wu
XGboost is a tree-based algorithm in the supervised branch of Machine Learning. Unlike Gradient Boosting, XGBoost builds trees using the similarity score and Gain to determine the best node splits. Step 1: Input dataset {xᵢ, yᵢ} from i = 1 to n = 4. Step 2. We assign the initial prediction as 0.5. 0.5 is the default value in the XGBoost module. Thus, the initial residual is the difference between observing y and y_initial. Step 3.
Try JournoFinder For Free
Search and contact over 1M+ journalist profiles, browse 100M+ articles, and unlock powerful PR tools.
Start Your 7-Day Free Trial →