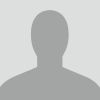
Hongming Li
Articles
-
Jan 25, 2025 |
biorxiv.org | Hongming Li |Zaixu Cui |Matthew Cieslak |Taylor Salo
AbstractThe brain functional connectome development is fundamental to neurocognitive growth in youth. While brain age prediction has been widely used to assess connectome development at the individual level, traditional approaches providing a global index overlook the spatial variability and inter-individual heterogeneity of functional connectivity (FC) development across the cortex. In this study, we introduced a regional brain development index to assess spatially fine-grained FC development.
-
Dec 11, 2024 |
biorxiv.org | Hao Zheng |Xiaoyang Chen |Hongming Li |Tingting Chen
AbstractDeep learning-based cortical surface reconstruction (CSR) methods heavily rely on pseudo ground truth (pGT) generated by conventional CSR pipelines as supervision, leading to dataset-specific challenges and lengthy training data preparation. We propose a new approach for reconstructing multiple cortical surfaces using weak supervision from brain MRI ribbon segmentations.
-
Apr 29, 2024 |
biorxiv.org | Yuncong Ma |Hongming Li |Zhen Zhou |Xiaoyang Chen
AbstractPersonalized functional networks (FNs) derived from functional magnetic resonance imaging (fMRI) data are useful for characterizing individual variations in the brain functional topography associated with the brain development, aging, and disorders.
Try JournoFinder For Free
Search and contact over 1M+ journalist profiles, browse 100M+ articles, and unlock powerful PR tools.
Start Your 7-Day Free Trial →