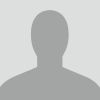
Ming Hu
Articles
-
Dec 6, 2024 |
mdpi.com | Ali Nouri |Ming Hu
All articles published by MDPI are made immediately available worldwide under an open access license. No special permission is required to reuse all or part of the article published by MDPI, including figures and tables. For articles published under an open access Creative Common CC BY license, any part of the article may be reused without permission provided that the original article is clearly cited. For more information, please refer to https://www.mdpi.com/openaccess.
-
Jul 8, 2024 |
nature.com | Ming Hu
AbstractChromatin spatial organization plays a crucial role in gene regulation. Recently developed and prospering multiplexed DNA FISH technologies enable direct visualization of chromatin conformation in the nucleus. However, incomplete data caused by limited detection efficiency can substantially complicate and impair downstream analysis. Here, we present SnapFISH-IMPUTE that imputes missing values in multiplexed DNA FISH data.
-
Jul 4, 2024 |
nature.com | Sadman Sadeed Omee |Nihang Fu |Rongzhi Dong |Ming Hu |Jianjun Hu
In real-world materials research, machine learning (ML) models are usually expected to predict and discover novel exceptional materials that deviate from the known materials. It is thus a pressing question to provide an objective evaluation of ML model performances in property prediction of out-of-distribution (OOD) materials that are different from the training set. Traditional performance evaluation of materials property prediction models through the random splitting of the dataset frequently results in artificially high-performance assessments due to the inherent redundancy of typical material datasets. Here we present a comprehensive benchmark study of structure-based graph neural networks (GNNs) for extrapolative OOD materials property prediction. We formulate five different categories of OOD ML problems for three benchmark datasets from the MatBench study. Our extensive experiments show that current state-of-the-art GNN algorithms significantly underperform for the OOD property prediction tasks on average compared to their baselines in the MatBench study, demonstrating a crucial generalization gap in realistic material prediction tasks. We further examine the latent physical spaces of these GNN models and identify the sources of CGCNN, ALIGNN, and DeeperGATGNN’s significantly more robust OOD performance than those of the current best models in the MatBench study (coGN and coNGN) as a case study for the perovskites dataset, and provide insights to improve their performance.
-
Jan 24, 2024 |
nature.com | Lei Jin |Thomas Lavin |Kelsey R. Babcock |Ming Hu |Hongkui Zeng |Mriganka Sur | +1 more
Correction to: Nature Neuroscience https://doi.org/10.1038/s41593-023-01545-8, published online 11 January 2024. In the version of the article initially published, in Figs. 1–6 the minus sign was missing from “Day −7” and has now been inserted in the HTML and PDF versions of the article.
-
Jan 11, 2024 |
nature.com | Lei Jin |Thomas Lavin |Kelsey R. Babcock |Ming Hu |Hongkui Zeng |Mriganka Sur | +1 more
AbstractRabies-virus-based monosynaptic tracing is a widely used technique for mapping neural circuitry, but its cytotoxicity has confined it primarily to anatomical applications. Here we present a second-generation system for labeling direct inputs to targeted neuronal populations with minimal toxicity, using double-deletion-mutant rabies viruses. Viral spread requires expression of both deleted viral genes in trans in postsynaptic source cells.
Try JournoFinder For Free
Search and contact over 1M+ journalist profiles, browse 100M+ articles, and unlock powerful PR tools.
Start Your 7-Day Free Trial →