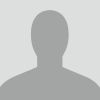
Qiaoling Wei
Articles
-
Jun 4, 2024 |
nature.com | Qiaoling Wei |Zhen Xing |Yi Lu |Chen Zhao |Weimin Tan |Bo Yan | +2 more
The transformative role of artificial intelligence (AI) in various fields highlights the need for it to be both accurate and fair. Biased medical AI systems pose significant potential risks to achieving fair and equitable healthcare. Here, we show an implicit fairness learning approach to build a fairer ophthalmology AI (called FairerOPTH) that mitigates sex (biological attribute) and age biases in AI diagnosis of eye diseases. Specifically, FairerOPTH incorporates the causal relationship between fundus features and eye diseases, which is relatively independent of sensitive attributes such as race, sex, and age. We demonstrate on a large and diverse collected dataset that FairerOPTH significantly outperforms several state-of-the-art approaches in terms of diagnostic accuracy and fairness for 38 eye diseases in ultra-widefield imaging and 16 eye diseases in narrow-angle imaging. This work demonstrates the significant potential of implicit fairness learning in promoting equitable treatment for patients regardless of their sex or age. Biased medical artificial intelligence systems pose significant potential risks to achieving fair and equitable healthcare. Here, we demonstrate a fairer ophthalmology AI that mitigates sexism and ageism through implicit fairness learning.
Try JournoFinder For Free
Search and contact over 1M+ journalist profiles, browse 100M+ articles, and unlock powerful PR tools.
Start Your 7-Day Free Trial →