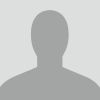
Zhen Xing
Articles
-
Aug 28, 2024 |
nature.com | Yupeng Zhang |Zhen Xing |Aijun Deng
Deep learning techniques were used in ophthalmology to develop artificial intelligence (AI) models for predicting the short-term effectiveness of anti-VEGF therapy in patients with macular edema secondary to branch retinal vein occlusion (BRVO-ME). 180 BRVO-ME patients underwent pre-treatment FFA scans. After 3 months of ranibizumab injections, CMT measurements were taken at baseline and 1-month intervals. Patients were categorized into good and poor prognosis groups based on macular edema at the 4th month follow-up. FFA-Net, a VGG-based classification network, was trained using FFA images from both groups. Class activation heat maps highlighted important locations. Benchmark models (DesNet-201, MobileNet-V3, ResNet-152, MansNet-75) were compared for training results. Performance metrics included accuracy, sensitivity, specificity, F1 score, and ROC curves. FFA-Net predicted BRVO-ME treatment effect with an accuracy of 88.63% and an F1 score of 0.89, with a sensitivity and specificity of 79.40% and 71.34%, respectively.The AUC of the ROC curve for the FFA-Net model was 0.71. The use of FFA based on deep learning technology has feasibility in predicting the treatment effect of BRVO-ME. The FFA-Net model constructed with the VGG model as the main body has good results in predicting the treatment effect of BRVO-ME. The typing of BRVO in FFA may be an important factor affecting the prognosis.
-
Jun 4, 2024 |
nature.com | Qiaoling Wei |Zhen Xing |Yi Lu |Chen Zhao |Weimin Tan |Bo Yan | +2 more
The transformative role of artificial intelligence (AI) in various fields highlights the need for it to be both accurate and fair. Biased medical AI systems pose significant potential risks to achieving fair and equitable healthcare. Here, we show an implicit fairness learning approach to build a fairer ophthalmology AI (called FairerOPTH) that mitigates sex (biological attribute) and age biases in AI diagnosis of eye diseases. Specifically, FairerOPTH incorporates the causal relationship between fundus features and eye diseases, which is relatively independent of sensitive attributes such as race, sex, and age. We demonstrate on a large and diverse collected dataset that FairerOPTH significantly outperforms several state-of-the-art approaches in terms of diagnostic accuracy and fairness for 38 eye diseases in ultra-widefield imaging and 16 eye diseases in narrow-angle imaging. This work demonstrates the significant potential of implicit fairness learning in promoting equitable treatment for patients regardless of their sex or age. Biased medical artificial intelligence systems pose significant potential risks to achieving fair and equitable healthcare. Here, we demonstrate a fairer ophthalmology AI that mitigates sexism and ageism through implicit fairness learning.
Try JournoFinder For Free
Search and contact over 1M+ journalist profiles, browse 100M+ articles, and unlock powerful PR tools.
Start Your 7-Day Free Trial →